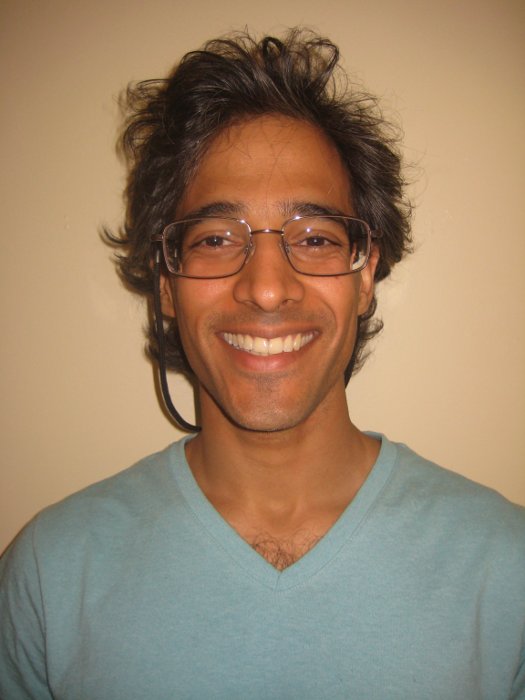
Aaditya Rangan
Associate Professor, Courant Institute, New York University and Flatiron Institute.
Date and time: May 8th, 2024 Wednesday, at 8am PDT / 11am EDT / 3pm BST / 5pm CEST / 11pm China
Ab-initio Reconstruction from small datasets: using entropy-maximization and principal modes (EMPM).
In this talk I’ll describe a simple algorithm for obtaining a low-resolution model from a small number (~1000) picked-particle images. The algorithm (termed EMPM) is similar to classical alternating-minimization, but with a slight twist: the entropy of the viewing-angle distribution is maximized during the search. This entropy-maximization greatly improves robustness and accuracy, allowing EMPM to outperform many existing ab-initio reconstruction algorithms. Because of its low computational overhead, this algorithm could facilitate statistical strategies such as bootstrapping and cross-validation, along with the rapid assessment of particle quality.
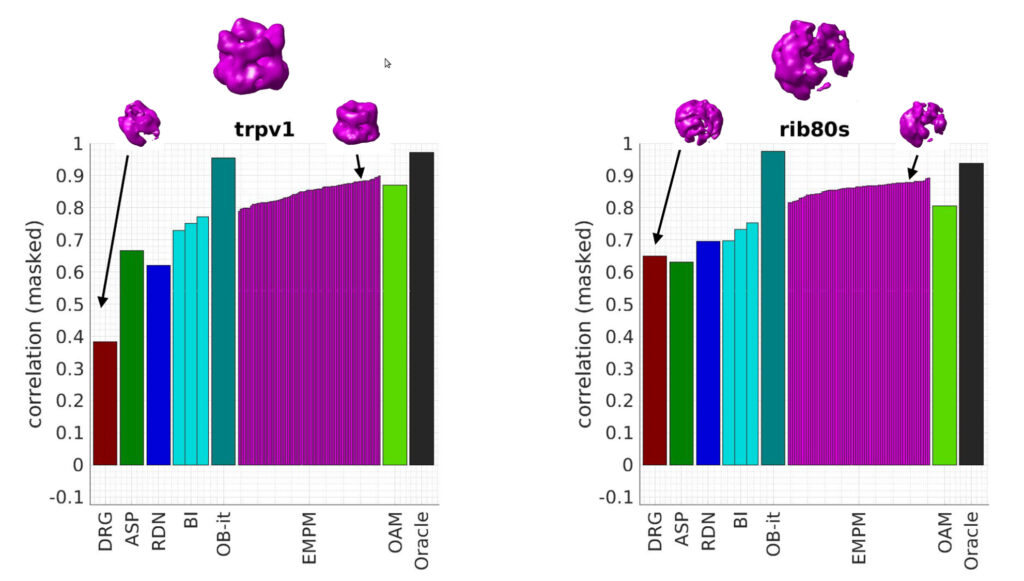