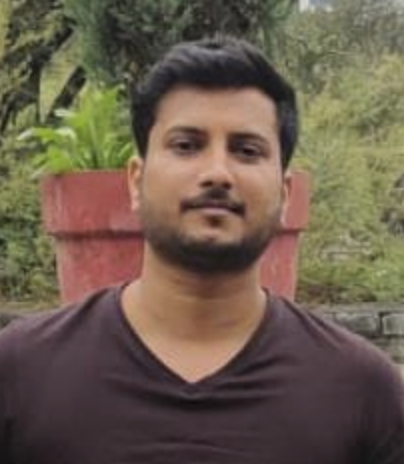
Harshit Gupta
Postdoc, Stanford Linear Accelerator Center (SLAC)
Date and time: November 10th, 2021
Wednesday, at 8am PT / 11am ET / 4pm GMT / 5pm CET / 12am China (November 11th)
For the zoom and gather.town links, please join the One World Cryo-EM mailing list.
CryoGAN: A New Reconstruction Paradigm for Single-Particle Cryo-EM via Deep Adversarial Learning
Cryo-electron microscopy (Cryo-EM) has revolutionized structural biology over the last decade by delivering 3D structures of biomolecules at near-atomic resolution. It produces many noisy projections from separate instances of the same but randomly oriented biomolecule. These noisy projections are then used to reconstruct the 3D structure of the biomolecule. Scientists have spent the better part of the last 30 years designing a solid computational pipeline to achieve this goal. The result is an intricate multi-steps procedure that permits the regular discovery of new structures, but that is yet still prone to overfitting and irreproducibility. The most notable difficulties with the current paradigms are the need for pose-estimation methods, the reliance on user expertise for appropriate parameter tuning, and the non-straightforward extension to the handling of biomolecules with multiple conformations.
CryoGAN is a new paradigm for single-particle cryo-EM reconstruction based on unsupervised deep adversarial learning. CryoGAN sidesteps the pose-estimation problem by using a generative adversarial network (GAN) to learn the 3D structure whose simulated projections most closely match the acquired projections in a distributional sense. The architecture of CryoGAN resembles that of standard GAN, with the twist that the generator network is replaced by a model of the cryo-EM image acquisition process. CryoGAN is an unsupervised algorithm that only demands projection images. No initial volume estimate or prior training is needed. CryoGAN requires minimal user interaction and can provide reconstructions in a matter of hours on a high-end GPU. In addition, it is backed by mathematical guarantees on the recovery of the correct structure. Moreover, its extension, called MultiCryoGAN can reconstruct continuous conformations of dynamic biomolecules, thus helping solve the most important open problem in the field without pose or conformation estimation.
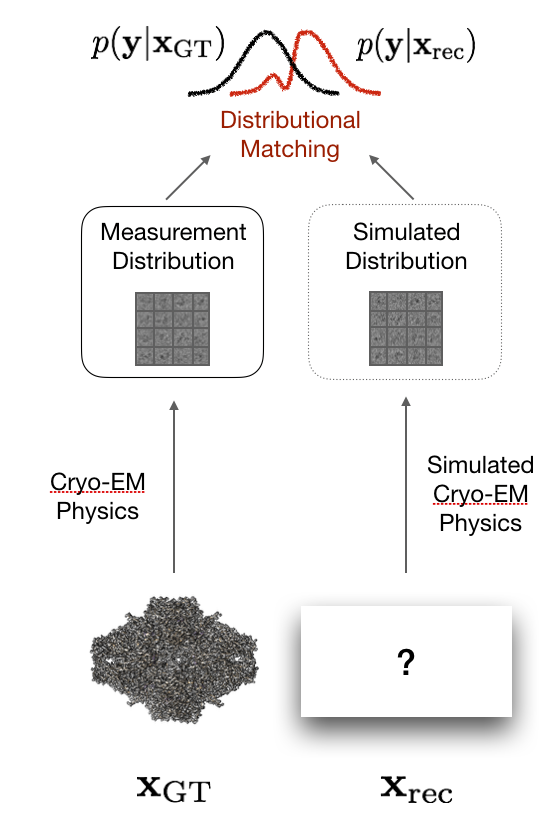