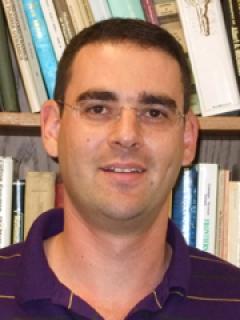
Amit Singer
Professor of Mathematics at Princeton University
Wilson Statistics: Derivation, Generalization, and Applications to Cryo-EM
Date and time: June 16, 2021
Wednesday, at 8am PT / 11am ET / 3pm GMT / 5pm CET / 11pm China
For the zoom and gather.town links, please join the One World Cryo-EM mailing list.
Note that we no longer use the zoom registration system, so old zoom links may not work. The new links will be sent via the mailing list.
The power spectrum of proteins at high frequencies is remarkably well described by the flat Wilson statistics. Wilson statistics therefore plays a significant role in X-ray crystallography and more recently in cryo-EM. Specifically, modern computational methods for three-dimensional map sharpening and atomic modelling of macromolecules by single particle cryo-EM are based on Wilson statistics. In this talk we provide the first rigorous mathematical derivation of Wilson statistics. The derivation pinpoints the regime of validity of Wilson statistics in terms of the size of the macromolecule. Moreover, the analysis naturally leads to generalizations of the statistics to covariance and higher order spectra. These in turn provide theoretical foundation for assumptions underlying the widespread Bayesian inference framework for three-dimensional refinement and for explaining the limitations of autocorrelation based methods in cryo-EM.