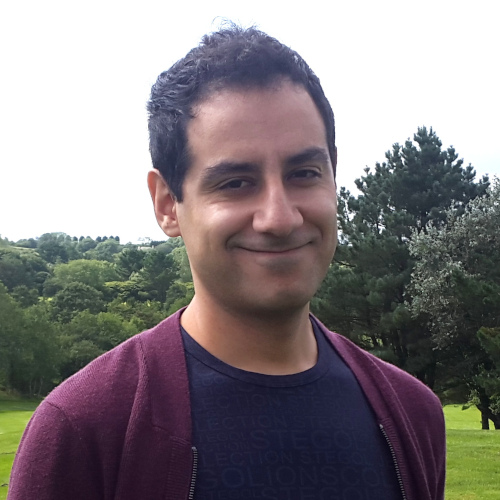
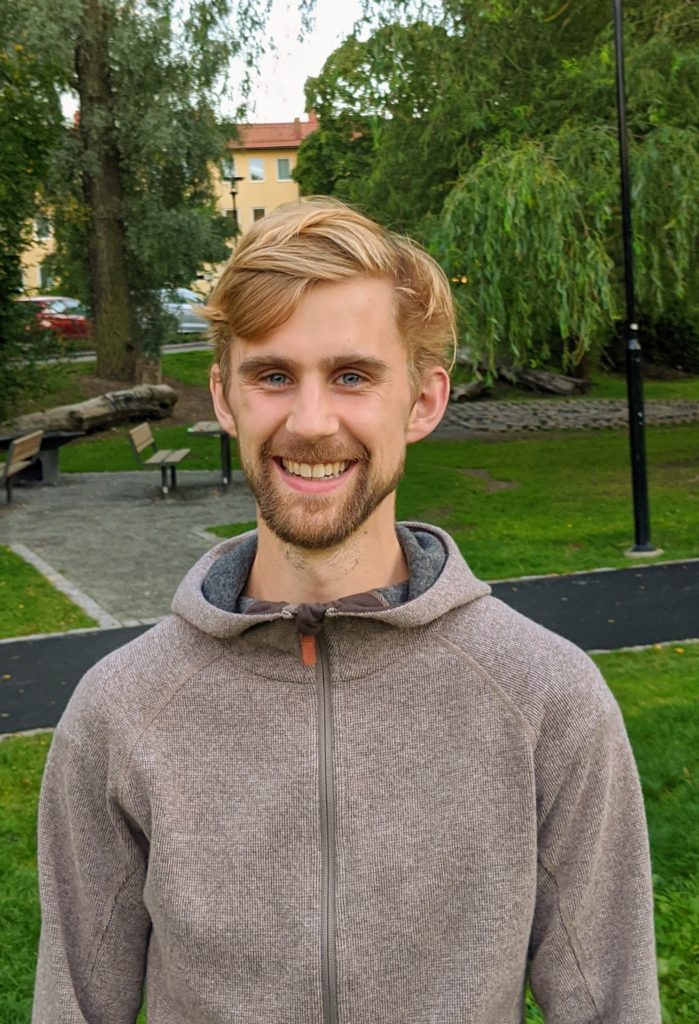
Dari Kimanius & Gustav Zickert
Dari Kimanius – Postdoc at MRC Laboratory of Molecular Biology
Gustav Zickert – Guest researcher at KTH Royal Institute of Technology
Cryo-EM structure determination with data-driven priors
Date and time: Feb 17th, 2021
Wednesday, at 8am PT / 11am ET / 4pm GMT / 5pm CET / Feb 18th midnight China
For the zoom and gather.town links, please join the One World Cryo-EM mailing list.
Note that we no longer use the zoom registration system, so old zoom links may not work. The new links will be sent via the mailing list.
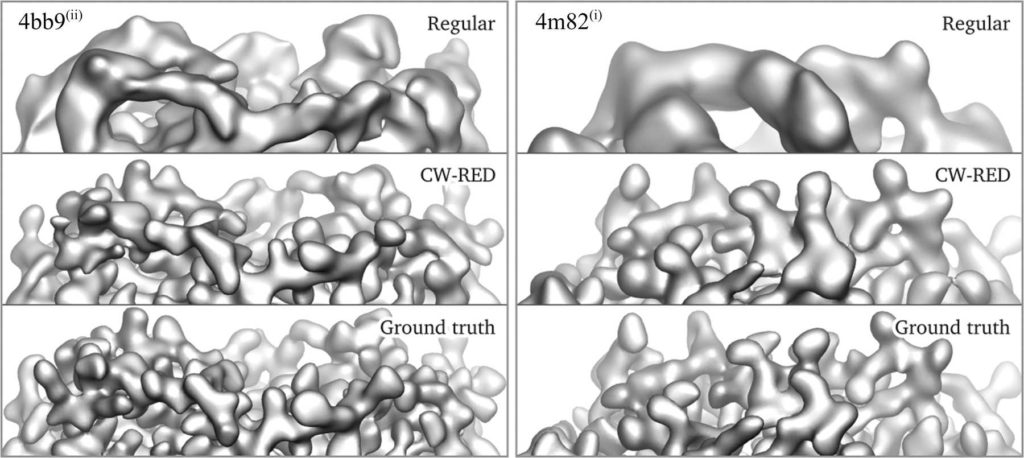
Three-dimensional reconstruction of the electron-scattering potential of biological macromolecules from electron cryo-microscopy (cryo-EM) projection images is an ill-posed problem. The most popular cryo-EM software solutions to date rely on a regularization approach that is based on the prior assumption that the scattering potential varies smoothly over three-dimensional space. Although this approach has been hugely successful in recent years, the amount of prior knowledge that it exploits compares unfavorably with the knowledge about biological structures that has been accumulated over decades of research in structural biology. Here, a regularization framework for cryo-EM structure determination is presented that exploits prior knowledge about biological structures through a convolutional neural network that is trained on known macromolecular structures. This neural network is inserted into the iterative cryo-EM structure-determination process through an approach that is inspired by regularization by denoising. It is shown that the new regularization approach yields better reconstructions than the current state of the art for simulated data, and options to extend this work for application to experimental cryo-EM data are discussed.